Machine intelligence is a mighty tool – at least for now. It is a tool that can help create better, smarter products. However, if UX designers and machine learning experts aren’t speaking the same language, they will hardly achieve any substantial results.
Machine learning concepts and language are anything but intuitive. Similarly, UX design requires comprehension of how users behave and think. UX design agencies consider the disorderliness of daily life and the irrationality of human behavior.
These two disciplines require different skills, so, usually, UX designers and machine learning specialists work in their respective areas, even though they could be creating the same thing. Frequently, professionals from both fields don’t know much about the methods and tools either of them uses. That is why they don’t usually see the potential in what they can achieve if they combine the two areas. To reduce these professional barriers, the product team must make a steady and deliberate effort. The question is: what do you start with?
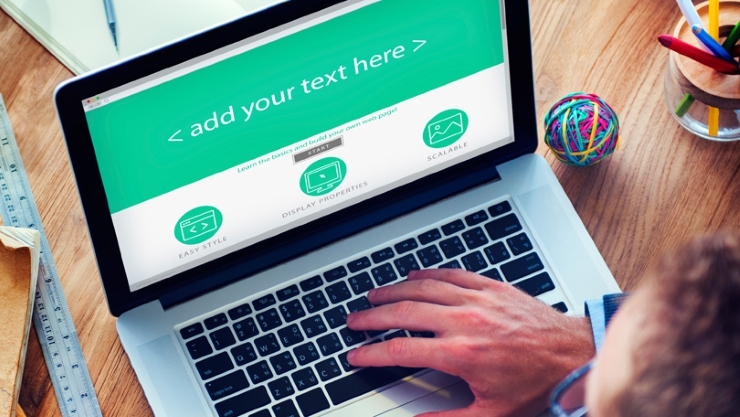
Below there are four vital principles for discovering an effective way of combining the most excellent UX design methods with machine learning techniques.
1. Develop a Common Language
The whole team should comprehend fundamental user experience problems, business goals, and a product’s vision. Your agency might be able to create an outstanding user experience in just one case – if the machine learning department and product design team are sharing information and ideas using a common language with concepts both of them understand.
The key to success here is machine learning specialists and UX designers joining forces to make a standard product development draft that includes data pipelines and user interfaces. A jointly created selection will provide your team with a clearer perspective regarding user experience in terms of how every solution you may provide in your design will affect the end-user experience. That, in turn, will help your team make informed decisions throughout the design process. One of the best ways to do this is placing both machine learning and UX design experts in the same workspace, so they can work side by side and share ideas, look for solutions together, and come to joint breakthroughs.
There are also two crucial questions, answers to which will help set up a universal language. The first of them is “Why?” Why did you select this particular machine learning or UX design solution for this specific situation? The second question is, “What is the goal?” What should happen after tuning a UX design detail or machine learning model optimization? For instance, each member of the team has to understand why writing an excellent copy in a notification can affect user engagement more positively, rather than carrying out machine learning model optimization for more accurate individual content recommendations.
2. Put the Use Case at the Center
In case you’re making a consumer product, you should primarily focus on the business’s goal and user experience you want to achieve, instead of technology.
Formulate and fix your use case. For instance, let’s say you are working on a personalized registration for a news application – your machine learning specialists and UX designers have to outline and then design the exact use flow for registration. This way, your entire team will see the main spots that can be improved by machine learning, thus boosting user experience. The same goes the other way around. Particular design solutions, including input from data scientists, data engineers, and designers, help set reasonable goals and expectations for the very first iteration of the product.
Proper use case comprehension allows the team to define an appropriate KPI for UX development that lines up with machine learning metrics. For instance, if you are developing AI-powered individual news notifications for a news app, the goal is to save the end-users time via automatic notifications. At the same time, you want to measure if app users like the notifications they see even without opening the app. You can track this by checking if the user keeps the smart notification function on or if they turn it off.
3. Mix Quantitative and Qualitative Data
Machine learning is not always about making use of “big data.” Moreover, historical data may even become an obstacle if you think that you can find answers to the UX design questions in the past quantitative data. Plus, you can take advantage of such things, like online learning, and avoid historical data altogether.
To comprehend the effects of UX design and machine learning combination, you will need both quantitative and qualitative data. Qualitative research methods, such as user testing, user interviews, and questionnaires, will help you understand how users perceive the features of the product you design – it helps seeing how users behave with the product. Thereby, qualitative data provides clarity on such things, like feelings and thoughts of end-users regarding the product and its features.
When working on a new project, you may run into a lot of unexpected factors that affect machine learning development and user experience design. Does a chosen data point capture the intention or the actual user behavior? Why doesn’t the feedback loop collect meaningful data – is it due to the inaccessibility of the UI feature or something else? The blend of quantitative and qualitative methods provides a broader perspective when it comes to such questions.
Also, user tests and interviews enliven the data. They emphasize the authentic connections between the users and the ways your system interprets them. A comprehensive understanding of the end-user is critical in singling out the signal from all the noise received in the data flow. Blending insights set up on both quantitative and qualitative data allows machine learning specialists and UX designers to better comprehend the product in its entirety as a part of regular people’s daily lives. In other words, each team member slowly becomes a product expert.
4. Align Your Decisions with Actual Data in Real-Life Environment
Will the user appreciate a smart assistant that can order food, manage a PayPal account, or book a hotel totally on its own? How can you make sure that artificial intelligence is employed to create smooth and coherent experiences for the user?
By creating a complex functional solution, you get a bigger picture of how machine learning and user experience fit together in reality. A minimally viable product that has operative machine learning models and data pipelines simplify product iteration and enable direct feedback from users via beta tests and user tests. This feedback should be shared, discussed, and deeply analyzed by the entire team. As a result, you will see how the product works in the real environment and determine the most vital things for further product development.
The Two Sides Working for the Common Result
When both machine learning specialists and UX designers understand product development problems, product development goes faster. The entire process generally becomes more productive. In the meantime, your designers, data scientists, and data engineers get fresh insights on the way machine learning could be employed for a better understanding of human behavior, as it doesn’t always follow the formulae, models, and predicted patterns. Also, UX designers get a better idea of how machine learning can be put to use for better user experience and, thus, better products.